What is the Poisson Dilemma?
Single-cell screening relies on microfluidic droplet encapsulation to isolate and analyse individual cells. However, one of the fundamental challenges in this process is the randomness of droplet content distribution, governed by Poisson statistics. This randomness often results in empty droplets or droplets containing multiple cells, leading to inefficiencies and data variability. Addressing this challenge is crucial for improving the reliability and accuracy of single-cell functional analysis.
The Problem with random droplet distribution
In traditional droplet microfluidics, cell encapsulation follows a Poisson distribution, meaning that many droplets remain empty, while others contain multiple cells. This inefficiency creates several issues:
- Wasted resources: Empty droplets consume reagents and chip space without contributing useful data.
- Cross-contamination: Multiple-cell encapsulation can introduce signal interference and confound downstream analysis.
- Low sequential assay success rate: The probability of correctly pairing specific cells, molecules, or reagents within droplets is significantly reduced.
Lightcast’s solution: Machine learning-based droplet filtering
To overcome the limitations of Poisson-driven randomness, Lightcast integrates machine learning algorithms to actively filter and enrich for single-cell occupancy. This innovation brings several advantages:
- Enhanced Single-Cell Encapsulation: By selectively removing unwanted droplets, the system increases the proportion of single-cell droplets to over 90%.
- Reduced Experimental Waste: Filtering out empty or incorrectly populated droplets optimises reagent use and chip space.
- Improved Sequential Assays: A controlled distribution ensures that critical cell-cell or cell-molecule interactions occur as intended, boosting the reliability of functional assays.
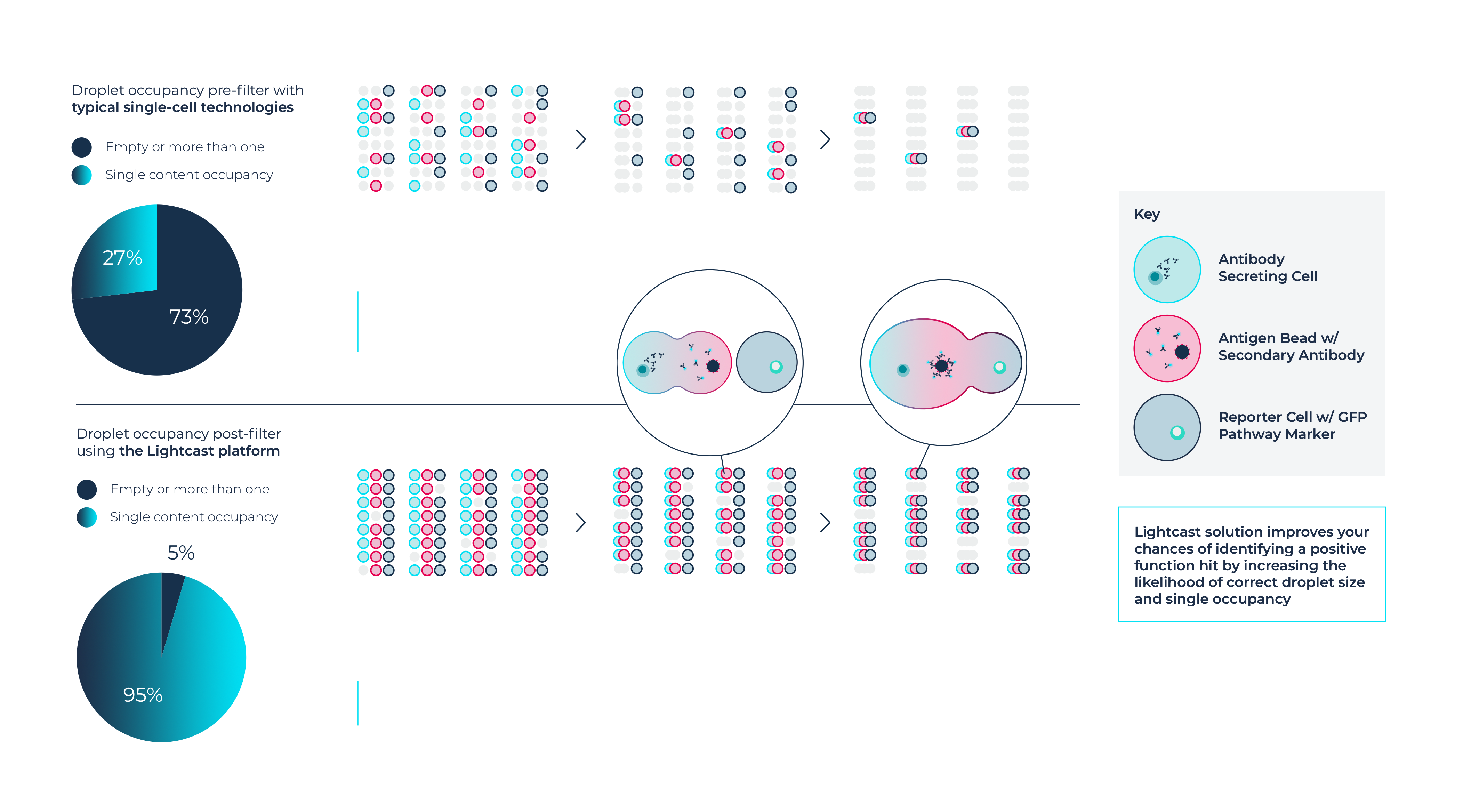
Turning droplet encapsulation randomness into reliable functional screening
By mitigating randomness in droplet encapsulation, Lightcast’s solution enhances the efficiency and accuracy of single-cell analysis. This translates into:
- Higher throughput: More meaningful data points from each experiment.
- Better reproducibility: Improved consistency in functional screening results.
- Faster drug discovery timelines: Reducing inefficiencies accelerates the identification of promising therapeutic candidates.
The challenge of random droplet content distribution has long hindered the potential of single-cell technologies. By leveraging machine learning to actively filter and enrich for properly encapsulated cells, Lightcast addresses this critical bottleneck, paving the way for more reliable functional screening. This innovation ensures that every droplet contributes valuable insights, ultimately accelerating advancements in drug discovery.
Download to full document